This in depth text will show that the methods used in virology are not valuable in proving the existence of a virus and are certainly not indicative of a true isolation of viral particles or genetic material. This text does not pertain to that of bacteria, fungus or parasites, all of which are not the cause of illness, but rather are a part of the delicate bioremediation and healing of the body. Elaboration on those topics will occur in other posts.
The scientific definition of the word isolate from Oxford is “obtain or extract (a compound, microorganism, etc.) in a pure form”. The definition of pure from Oxford is “not mixed or adulterated with any other substance or material”. However, admittedly in virology, it is understood that a virus is a replication-competent particle. It requires host cells or tissues to ‘exist’. Therefore, it is impossible to isolate a virus in the truest sense of the word.
Virologists consider isolation to be the virus cultured in a cellular medium. This text will begin with a small section on why viral isolation is impossible, the virologists’ use of the word isolate, and the process of ‘isolation’, realistically known as culturing the virus. Largely this post is concerned with assessing the existence of a virus, as it is impossible to isolate it. Thus, we will explore the methods used that ‘confirm’ the existence of a virus in a cell culture, as well as in clinical or environmental samples. There are four ways to confirm the existence of a virus, since we cannot rely on Koch’s or Rivers’ postulates, as they require an isolated virus. We will call them the four horsemen of virology: cytopathic effects, electron microscopy, immunological methods, and nucleic acid detection.
This text will show the recurring circular reasoning and confirmation bias consistent throughout virology. This illogical reasoning is what convinces those studying this matter that they are onto something. But there is no starting point. No isolated and purified virus. No isolated and purified genome. Therefore, no isolated variables, no scientific methodology used. These methods also lack valid controls. The definition of pseudoscience from Oxford:
a collection of beliefs or practices mistakenly regarded as being based on scientific method.
Viral Isolation (Cell Culture)
Let’s explore the steps used in the isolation of a virus, more realistically known as the viral culture. Viral culture is known as the gold standard method of identifying unknown viruses. The purpose of culturing a virus is to concentrate the virus. Cell cultures allow for the amplification of the virus. The linguistics a virologist will use is that cell cultures allow the isolation of viruses from clinical or environmental samples.
First, we must collect appropriate samples from the patient or environment, such as swabs, fluids, tissues, feces, etc. The sample type depends on the suspected virus and the pathogenesis of the infection (e.g., respiratory, gastrointestinal, neurological). Next, we must cultivate the viruses. So, we inoculate the clinical samples onto susceptible cell culture systems. The virus will replicate in the cell culture. Specialized cell lines or culture conditions may be required for different viruses. Within this cell culture, we have cell culture medium (Vero cells, MDCK cells, etc.), fetal bovine (or other animal) serum, antibiotics, antimycotics, protease inhibitors, growth factors, cytokines, pH indicators, pH buffers, etc.
Et voilà, you have isolated a virus, by the standards of virology. Believe it or not, that is the process of viral isolation. Thinking back to our definitions, we understand that this is not a true isolation of a virus. Now, how do we know that the virus is present in this sample? We get back to the four methods of assessing the presence/existence of a virus, namely cytopathic effects, electron microscopy, immunological methods, and nucleic acid detection.
Cytopathic Effects
Viruses can be detected by their ability to infect and kill cells in culture. Cytopathic effects (CPE) are changes in infected cells compared to uninfected controls, commonly known as mock infection control groups. CPE refers to the morphological changes and damage that occur in host cells as a result of viral infection. Viruses that cause degeneration of the host cells are considered cytopathogenic. Common CPEs include cell rounding, swelling, shrinkage, lysis (cell death), formation of syncytia (multinucleated cells), and appearance of intracellular inclusion bodies.
CPEs are observed by virologists, often through a light microscope. Now, virologists use automated image analysis tools like the Celigo Image Cytometer that can rapidly scan entire well plates (96 wells or 384 well plates), identify infected cells, and quantify CPE metrics. The system uses proprietary image analysis algorithms optimized for virology applications to quantify CPE. This automated imaging eliminates the need for tedious microscopy. There are a few ways to measure and quantify CPE, namely TCID50 and Plaque assays.
The Tissue Culture Infectious Dose 50 (TCID50) assay is a method used to quantify the amount of virus in a sample. TCID50 is defined as the dilution of a virus that will infect 50% of the cell culture inoculated with that dilution. It is an endpoint dilution assay expressed as TCID50 per unit volume (e.g., TCID50/mL). The TCID50 assay involves infecting a series of cell culture wells with different dilutions of the virus sample, then observing the wells for the development of cytopathic effects (CPE) or other signs of viral infection. Several statistical methods can be used to calculate the TCID50. The widely used and accepted methods have been criticized for introducing bias. Traditional TCID50 assays rely on the subjective visual assessment of CPE in cell cultures, which can introduce variability. Virus titers often exhibit significant variability, in part due to the inherent biological variation present in living systems, and also because they are based on serial limiting dilution data, which is known to be a source of error. This is another major source of variability. The key limitations of the TCID50 assay include biased calculations, incorrect assumptions, reliance on specific infection patterns, and most notably, subjectivity in CPE detection.
The other method, the plaque assay, is used to determine the concentration of viruses or bacteriophages. This paragraph will discuss this method in relation to viruses. Plaque assays involve infecting a monolayer of host cells (e.g., mammalian cells) with dilutions of a virus sample. When a virus infects and lyses a single host cell, it creates a visible “plaque” – a cleared area in the otherwise confluent cell monolayer. Each plaque represents the result of a single infectious virus particle, known as a plaque-forming unit (PFU). By counting the number of plaques at the appropriate virus dilution, researchers can determine the concentration of infectious virus particles in the original sample. Plaque assays are considered the “gold standard” for quantifying virus titers, as they directly measure the number of infectious virions. The quality of the plaques formed can be variable, with some appearing small or difficult to count accurately. This can introduce subjectivity and reduce the precision of the plaque count. Again, virus titers often exhibit significant variability, in part due to the inherent biological variation present in living systems, and also because they are based on serial limiting dilution data, which is known to be a source of error.
Both methods, namely TCID50 and Plaque assays, are controlled in the same manner. This control is known as the mock infection control or mock-infected cells, which refers to the control group or sample that is treated the same as the experimental group exposed to a virus but is not actually infected with the virus. The purpose of using a mock-infected control is to isolate the specific effects of the virus from other factors that may influence the experimental results. In this case, the mock-infected control group would be subjected to the same cell culture conditions, media, incubation times, etc., as the virus-infected cells but without exposing them to the virus. This allows the researchers to distinguish the virus-specific effects on the cells from any non-specific impacts of the experimental setup.
Comments:
The major problem with the study of cytopathic effect, and virology in general, is the mock infected groups. When reading the literature, you’ll notice that the methodology involved in setting up the mock infected groups is completely omitted. Not only is this bad science, but it is also very misleading. We assume that the mock infected groups follow the general understanding of mock infection, as there is a plethora of information on the concept of mock-infection, but in practice, it is not utilized properly and in conjunction with the definitions attributed to it. Based on the track record of virology and their loose use of isolation, I don’t think the field merits our trust to assume that the use of mock infection in a methods section refers to the proper isolation of the virus and control of the cell culture parameters. Not one paper has ever described their methods used in setting up a mock infection, any more than naming their control group a “mock infection”.
Mock infection should control all the introduced variables in the cell culture, namely fetal animal serum, antibiotics, antimycotics, protease inhibitors, growth factors, cytokines, pH indicators, pH buffers, etc. In reality, mock infections are just cells and nutrients. This is what you call a trick of the trade. There is a reason that the methods involved in describing a mock infection are omitted from the study parameters, as instead of lying, they are just not telling the truth. But again, this is just bad science. It is misleading, non-transparent, doesn’t allow for the true reproducibility of the study, and goes against true science.
There is also the concept of cell starvation. One approach virologists use is to starve the cell culture, to increase the uptake of virus. An equally valid interpretation could be that starvation is causing cellular breakdown. But as per usual, this is inconceivable, and through argument from incredulity, we deem it impossible. Furthermore, the addition of antibiotics and antibiotics and antimycotics are to control and exclude the option of the cells being killed by bacteria or fungus. This is another tricky area. Virologists will be convinced of their controls because they are controlling the variable of bacterial or fungal cause of death. But in reality, they are adding reagents that are going uncontrolled.
The claims made in this section are backed up but some very compelling evidence. Dr. Stephan Lanka controlled this process and was able to produce viruses in cell cultures without the addition of virus. He showed micrographs of the cell cultures, the control group 1 contained freshly isolated tissue with low amounts of antibiotics/antimycotics with healthy cells, control group 2 contained low amounts of antibiotics and antimycotics, 10% fetal bovine serum and Dulbecco’s Modified Eagle Medium (DMEM), experimental group 1 contained normal amounts of antibiotics and antimycotics, 1% fetal bovine serum and DMEM (standard viral culture procedures in CPE studies), and group four was set up the same with the addition of yeast RNA. In the experimental groups cell death was observed as plaque formation. No virus was added in any of his groups, yet he observed CPE.
If anything can be drawn from Dr. Lankas experiments is that the mock infection process is a very delicate and specific process. The process of mock infection should be specifically described in papers, but I have yet to find a paper that describes their exact methodology other than creating a mock infected control. If healthy cells that are well fed and have low quantities of antibiotics and antimycotics are used as the control, this is completely invalid and makes no sense for the experiments occurring. The driving factor of cellular break down or CPEs are due to the addition of the chemicals and starvation. These experiments are being recreated, and furthered by Mr. Jamie Andrews.
Based on this information, the methods of assessing cytopathic effects are null and void. Microscopy or automated visualization is redundant. TCID50 and Plaque essays are whimsical. Even in the foundational papers explaining the methodology and statistical analysis behind TCID50 and plaque assays, the mock infected/control groups are not described, the papers state solely ‘mock infected control’. These methods are meant to woo the scientists with numbers and data, when the fundamentals of the cell culture are uncontrolled.
CPEs are just cell death. Cells are dying from starvation or poisoning. What causes the cell death is completely uncontrolled in these experiments, and when controlled, and objectively assessed, it is obvious that the CPEs are caused by the chemicals in the cell culture and the lacking nutrients, and not any exogenous virus. Confirmation of the virus being present in the cell culture relies on the other three methods, namely electron microscopy, immunological methods, and nucleic acid detection.
Electron Microscopy
An often argument in favour of the existence of viruses is that we have pictures of viruses. However, images by electron microscopy (EM) do not follow the scientific method. It is not necessarily useless, but it is uncontrolled, and makes many assumptions. To draw conclusions from images is unscientific enough, as the full picture is not observed, we only see a snapshot. Any conclusions and inductions from EM would be considered pseudoscientific, as the method doesn’t employ a control. Were going to explore the three types of EM, namely Transmission EM (TEM), Cryo-EM, and Scanning EM (SEM).
Transmission Electron Microscopy
Let’s first explore the process of Transmission Electron Microscopy (TEM). We will first explore the process objectively, then I will provide some comments on the process and methodology.
Process of Transmission Electron Microscopy
First, we must prepare the sample. The target virus from a cell culture is used to obtain a high number of viral particles. A sample of this culture is fixed using appropriate chemical fixatives to preserve the ultrastructure. Next, the sample is dehydrated through a graded series of ethanol or acetone solutions. Then, we embed the sample in a resin, such as epoxy or acrylic resins, to provide support, essentially replacing the cytoplasm that was dehydrated. An ultramicrotome is then used to cut the embedded sample into ultra-thin sections, typically 50-100 nm thick. Now, the sample is ready for mounting. Place the thin sections onto a copper or nickel TEM grid, which has a mesh-like structure that provides support and allows the electron beam to pass through.
Once mounted, the next step involves staining the sample. We must stain the sections with heavy metal compounds, such as uranyl acetate and lead citrate, to increase the contrast of the sample. The heavy metals selectively bind to certain cellular components, enhancing their visibility under the electron beam. Lastly, we visualize the sample. Here, we focus the electron beam on the sample, which in turn creates an image.
Comments:
First, I want to highlight the point that this entire process is an uncontrolled endeavor. The steps involved in setting up a slide for TEM cannot be controlled as they are necessary for visualization. It is impossible to control this endeavor, making it an unscientific tool, in which no conclusion, inference, or induction should be conducted, and considered scientific.
Considering the literature, we understand now that the method of setting up a cell culture creates a ‘virus’ as an artifact. The ‘mock infected control’ is an invalid control, as all the steps in the cell culture are accounted for and controlled. Therefore, when you see TEM pictures in the literature of the mock infected group vs the experimental group (cell culture), the mock infected group shows no particles as the mock infect group is just cells, and the cell culture, which contains the plethora of chemicals alongside the so-called ‘virus,’ shows particles.
An argument for TEM is that the same images show up every time. Here it is important to not conflate consistency with accuracy. Consistency doesn’t mean the process is correct. Just because the process produces similar results, doesn’t mean that it is a correct process. If I am trying to hit a target, but I am hitting it 10 inches wide every time, I am consistent, but not accurate. Accuracy must be proven alongside consistency for something to be a valid tool/method. An explanation for its consistency is that the same method of setting up a slide is used every time TEM is conducted. The cells are altered in the same fashion and order, leading to the same images.
I will reference the assumptions made when utilizing TEM in an experiment, highlighted by Harold Hillman. This is largely concerned with that of cell biology and the fallacies thereof, but it translates to the entire process of TEM in general. The first assumption is that the effects of freezing, e.g., shrinkage and intracellular crystallization, do not produce irreversible changes in tissue. We also assume different parts of the tissue are dehydrated equally. Another assumption is that there are no significant structures which dissolve or diffuse away in the reagents. We assume that there are no significant structures which are not stained, that the organelles originally are equally hydrated in vivo and will shrink proportionately, that the heat and irradiation cause no significant change in the size or shape of the organelles, that the similarity of electron micrographs from subcellular fractions, fresh tissue, and fixed tissue means that electron microscopy has no effect on the preparation, that the ability to distinguish organelles clearly on electron micrographs is evidence of their biochemical viability before fixation.
Specifically concerning viruses, there are many more problems and limitations. Preparing viral samples for TEM, such as viruses, requires extensive and complex sample preparation steps like fixation, dehydration, embedding, and ultrathin sectioning. These sample preparation steps can introduce artifacts and may not preserve the native structure of the virus particles. TEM is not well-suited for quantifying viral titers or infectivity, as it does not provide a direct measure of the number of infectious virus particles. Other methods like plaque assays and TCID50, as mentioned above, are more commonly used to quantify viral infectivity.
TEM in and of itself is a rather subjective procedure as well. Which images are published? How many times are you allowed to run a TEM to get the desired pictures? Which images are omitted? Of course, you can see how obtaining the desired results would be rather easy, running the visualization process over and over. Are we supposed to trust the integrity of the scientists? There should be no trust involved in science. Trust is a rather unscientific term. Science is admittedly laden with fraud, vested interests, reproducibility limitations, dogma, etc. But I digress.
More on subjectivity, TEM cannot differentiate between infectious and non-infectious viral particles, as it only provides information about the morphology and structure of the viruses, again leading to the fact that TEM relies on other means of proof. Also, the morphological features of viruses are similar to subcellular structures, making the process obscure, of accurately identifying the specific viral species based solely on TEM observations. A paper by Akilesh et al., (2021) highlights some confusing aspects of viral identification using EM.
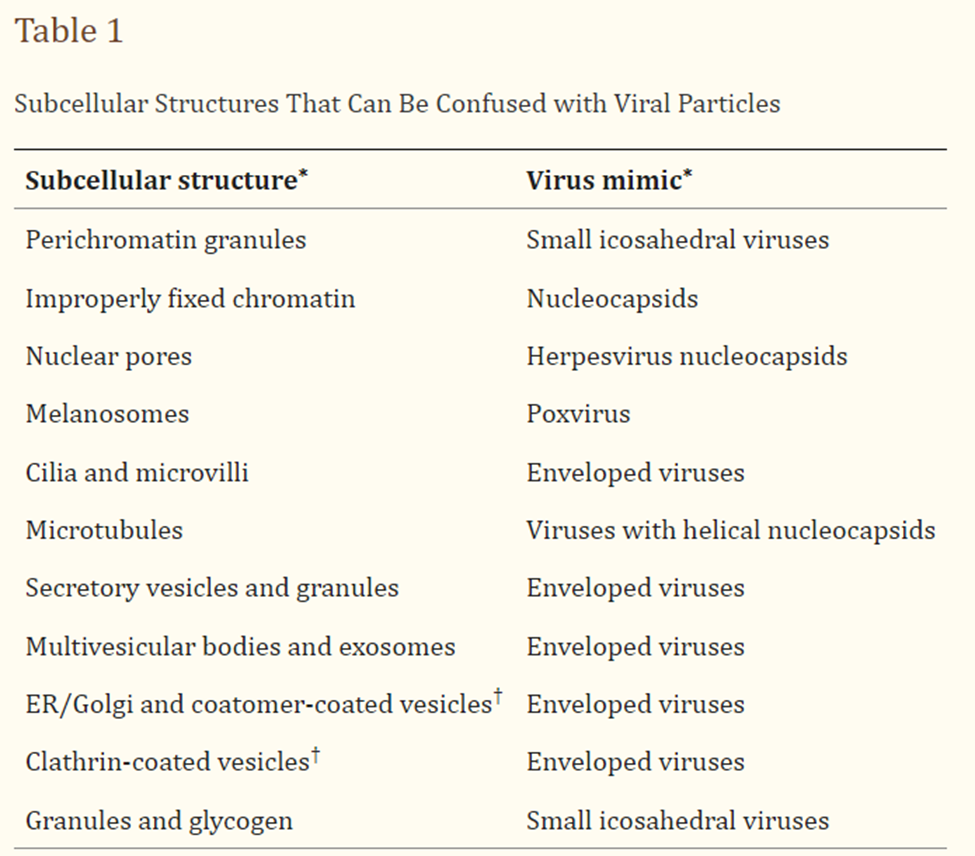
Image retrieved from here.
Regarding subjectivity, TEM images of cell cultures containing ‘virus’, or even clinical tissue samples from sick individuals (from ‘infectious diseases’) show similar particles as tissue samples from cancer patients, or other degenerative and chronic diseases. It is impossible to distinguish between ‘secretory vesicles’ and ‘viruses’ based on EM alone. I strongly suggest spending some time looking at TEM images of cancer patients, and of patients with other diseases other than ‘viral’ ones.
If we’ve learned anything from this section, it is that it is certainly not scientifically valid to rely solely on TEM to identify viruses, categorize viruses, determine pathogenicity, infectious nature, or realistically, anything about viruses. EM relies on the other three methods, namely, cytopathic effect, immunological tests, and nucleic acid tests to justify its claims.
Cryo Electron Microscopy
Some may point to Cryo Electron Microscopy and say we have isolated pictures of viruses. Many of the same principles highlighted in the last section on TEM are still relevant here, such as drawing conclusions about function and causation from images, etc. For completion, we will go over this process objectively, followed by some of my comments.
Process of Cryo Electron Microscopy
First, we must purify the viral particles and suspend them in an appropriate buffer solution. Here, we clarify the virus-containing cell culture medium by low-speed centrifugation or filtration to remove cell debris and large aggregates. Then, we concentrate the viral particles using techniques like polyethylene glycol (PEG) precipitation or sucrose cushion/density gradient centrifugation. In brief, PEG precipitation involves introducing PEG to the clarified medium, incubation, and pelleting the virus particles by centrifugation. The sucrose cushion requires layering the clarified medium over a sucrose cushion and ultracentrifuging the sample to pellet the virus.
Next, we must resuspend the purified viral pellet in an appropriate buffer solution. Here, the buffer can alter the structure of the particles, so caution should be taken when resuspending the viral pellet. It would be appropriate to assess the quality and concentration of the purified viral sample using techniques like plaque assay or negative-stain EM.
We then add a small volume of the viral sample we just described onto a grid with a thin carbon film containing holes. We must rapidly freeze the grid in liquid ethane or propane to vitrify the sample and prevent ice crystal formation. Next, we obtain the 2D images from the Cryo-EM machine. In good fashion, we use computers to generate 3D images. These are often created using other methods such as immunochemistry and the genetic sequences obtained computationally as well.
Comments:
Admittedly, the process of virus purification is most compelling in cryo-EM. It’s a shame that it’s the only method that uses this degree of purity. Let it be known that viral samples are also inherently heterogeneous; they contain a mixture of different viral particle conformations, assembly states, and potential contaminants. It is not really true purity, as cryo-EM wouldn’t show smaller particles or chemical contaminants.
The process of cryo also has many limitations, a big one being that not all virus types are capable of being purified to the degree needed for cryo. Viral proteins embedded in lipid membranes cannot be purified and maintained in their native conformation. The presence of the membrane can also complicate image processing and interpretation of the resulting structures. Additionally, smaller viruses (< 50 nm) are more challenging to image and reconstruct due to the limited number of particles and lower signal-to-noise ratio.
More limitations include the inability to capture the structural diversity within a viral species and an inability to differentiate structural features. It’s a subjective comparison. The structural differences between Virus-like particles (VLPs) and viruses cannot be distinguished. Based on images, you also cannot determine if it is a virus or a host cell vesicle, as viruses hijack host cell properties and are visually similar in nature. It is also impossible to directly link the observed structural features to the specific biological functions of the virus.
Additionally, cryo-EM captures static snapshots. It is impossible to reliably and truthfully induce any information about the interactions between viruses and their host cells, such as processes like viral entry, assembly, and maturation. Like TEM, cryo requires other confirming measures to ensure any conclusions drawn.
Scanning Electron Microscopy
Scanning electron microscopy (SEM) has also been used to show viruses on the surfaces of cells. At this point, the explanation is getting redundant so I will keep it short and meaningful. In SEM, a focused electron beam scans the surface of a sample and provides information about the surface composition of a sample. In this case, we are observing the outer portion of a cell, often one experiencing cytopathic effects.
To cut to the chase, there is no valid control. Mock infected groups are not properly set up to control the cell culture procedure properly. As Lanka’s results show, a poorly controlled mock infection produces VLPs and cytopathic effects, even when no virus is introduced.
What happens in studies that show SEM images of cytopathic effects in cell cultures, compared to healthy and well-maintained cells. None of the variables of the cell culture is controlled, leading to a completely fraudulent set of data. Again, ignoring the scientific method. The controls in these SEM studies show a relatively smooth surface of the cell with little VLPs, while the experimental groups show many VLPs at the cells surface.
Again, induction from these images would be completely biased and based on the current scientist’s worldview, namely based on the story of viral infection. We can not decipher any information of the particular virus, whether it is infectious, the species, the variant, or whether it is actually a virus and not a natural product of the host cell. No conclusions can be drawn from images about function or process. All EM relies on other methods to confirm findings.
Gold Standard Tests
I will now take a moment to discuss how tests are evaluated for efficacy and reliability. You often hear the term gold standard thrown around. A gold standard test is a diagnostic test that is considered the definitive, most accurate, and authoritative method for establishing the presence or absence of a particular condition or disease. An ideal gold standard test would have 100% sensitivity (identify all individuals with the disease, no false negatives) and 100% specificity (no false positives). In practice, there are not truly “perfect” gold standard tests, but the gold standard is the best available method that is as close to the ideal as possible. The gold standard may change over time as new and more accurate diagnostic methods become available. The performance of a new diagnostic test is evaluated by comparing its results to the gold standard, or other methods, that we will highlight further in this post. That determine sensitivity and specificity when no gold standard is available. Essentially this allows the assessment of a new test’s sensitivity, specificity which are fundamental measures of a test’s accuracy.
Comments:
Gold standard is a misleading term thrown around to make the laymen think that the experts know what they’re doing. Especially when it is in regard to something that can not be confirmed by our empirical observations, i.e., our senses. Testing for a virus, antibody, or protein, are things that cannot be confirmed by observation. It can only be visualized through electron microscopy, which has many confounding variables, as covered above. The question arises, how can a gold standard be determined in the first place?
How to Create a Gold Standard Test
Here this section will consider creating a test that cannot be confirmed by empirical observations, which although would still depend on language and opinions, it would be the best method to create a gold standard. The test in that case would be referring to the presence of the empirical observation. Here we are discussing the development of diagnostic tests.
Method 1: Use a latent class analysis (LCA) approach.
This is a statistical modeling approach that can estimate the sensitivity and specificity of multiple tests without requiring a gold standard. It works by treating the true disease status, the actual presence or absence of the disease in an individual, as a latent (unobserved) variable and using the results of the various tests to estimate the test performance characteristics. The model makes assumptions about the relationships between the tests and the true disease status. By fitting the model to the observed test results, it can provide estimates of the sensitivity and specificity for each test, as well as the prevalence of the disease in the population.
Some LCA models, like the finite mixture (FM) model introduces additional latent classes to capture the subpopulations of definitively healthy and definitively diseased individuals, in addition to the classes representing the true underlying disease status. The “definitively healthy” class include individuals who consistently test negative, regardless of the test used. A “definitively diseased” class include the individuals who consistently test positive, regardless of the test used.
LCA models are based off indicators. Indicators include, but are not limited to, the presence/absence of a symptom or condition, biomarker levels (e.g., blood pressure, cholesterol, HbA1c), severity of a condition, levels of patient-reported outcomes (e.g., quality of life), type of treatment received (e.g., medication, surgery, physical therapy), cause of death or primary diagnosis, nutrition, physical activity, obesity measures as well as maternal, infant, and child health outcomes. Essentially, assuming the disease manifestation of a disease state in an individual.
Which observed indicators to include in the model is a key decision. The adage of garbage-in-garbage-out holds. A clear rationale for the inclusion of any variable in the models should be presented, as observed indicators are the principal determinants of class characteristics. The indicators used for the analysis should, therefore, largely be dictated by the research question.
Quote retrieved from this paper.
Method 2: Compare the new test against a well-established diagnostic test
This approach involves using the results of the new test (T2) and comparing them to the results of an existing, well-established diagnostic test (T1) that does not necessarily have to be a gold standard. The apparent sensitivity (Se2,1) and specificity (Sp2,1) of the new test T2 can be calculated using the standard formulas:
Se2,1 = P(T2+ | T1+) & Sp2,1 = P(T2- | T1-)
However, since T1 is not a perfect gold standard, the true sensitivity (Se2) and specificity (Sp2) of the new test T2 need to be estimated using equations that account for the known performance of T1. This involves using the apparent test characteristics along with the sensitivity and specificity of the established test T1 to solve for the true Se2 and Sp2.
Method 3: Conduct a clinical impact study.
Instead of just evaluating diagnostic accuracy, this method assesses how the new test would impact clinical decision-making and patient outcomes compared to current practice. This can provide useful information about the test’s utility even without a gold standard. Notably, this is not a means to understand specificity and sensitivity.
Comments:
Considering method 1, of course we must highlight that there is an assumption made, that there is a relationship between a disease status (indicator), and a test. Which is not a small assumption. Moving past that, much must be assumed about the disease status as well, the nature of the symptoms, the diagnostic profile, etc. Symptom profile vary in each case. Each disease has a set of symptoms which may be present, and may not be present, which is the purpose of the development of tests. If diseases have specific symptoms, we wouldn’t need tests to diagnose them.
Confounding variables such as comorbid diseases must be considered in setting up this study as this can interfere with symptom profiles. Choosing the right indicators of disease is the fundamental testing unit of the model, a poor choice here could skew the results greatly! It is quite circular to employ a biomarker test that would not have a gold standard (PCR, antibodies, etc.) as the indicator.
There brings up another issue, namely the “definitively healthy” class and “definitively diseased” class are those that always test positive/negative on other tests. Thus this method still relies on other tests. Where is the starting point? Where is the ‘gold standard’ of test development?
There are many assumptions involved in this method. LCA models make assumptions about the relationships between the observed variables and the latent classes, as well as the distribution of the latent classes. Violations of these assumptions, such as local independence or correct number of classes, can lead to biased results and flawed interpretations.
Method 2: The major problem with Method 2 is that it relies on the results of method 1. Therefore, if method was done incorrectly, misinterpreted, or biased, the results of the comparison will reflect that of method 1. The apparent sensitivity and specificity calculated based on the agreement between the new and established tests may be biased, as the true disease status is unknown. This bias can then propagate to the estimated true sensitivity and specificity of the new test. This leads to conclusions about tests that have no foundation. One test may seem to be better than the other, but its all relative. Very easily can we slip into bias here.
Method 3: Seems like the most meaningful approach, however there are many presumptions within this method as well. I will reiterate that this is not a method to identify specificity and sensitivity, but a method to understand usefulness. Regardless, there is the assumption between the disease status (symptom profile, diagnostics) and the test. Choosing which marker/indicator to measure can greatly skew the results of this method as well.
Most notably, there is the assumption that a decrease in symptoms is indicative in healing when treated with an intervention. This is mistakenly taken as empirical evidence of healing. This is a confusing phenomenon, and you can understand the confusion. In theory a lack of symptoms is indicative of a healthy individual, however symptoms are not the cause of illness. There is an underlying cause of the disease, namely a toxicity, deficiency, or trauma. Suppressing symptoms does not remove the toxicity or nourish the deficiency. It disconnects our healing pathways (symptoms) leading to a facade of health.
As an example, I will highlight the broken finger. When you break your finger, inflammation occurs after the matter. The inflammation did not cause the broken finger. Reducing the inflammation does not repair the broken finger. The inflammation was the healing response the body had to increase blood flow, blood permeability, etc. The repression of symptoms is not indicative of healing. Any reasonable induction from this empirical observation would realize that all disease works this way. Considering ‘infectious diseases’ these are largely toxicity issues. Killing the microbe or reducing the symptoms do not remove the underlying toxicity, and further stores this toxicity.
Considering all three methods, there is the variability in the symptom profile in diseases. Often it is not enough to diagnose solely on our observations and symptoms, especially when it comes to very specific diseases. It is easy to diagnose the cold/flu, but impossible to diagnose someone for ‘covid’ vs ‘influenza’, or the strains thereof for that matter. Due to the variability in symptoms, we often employ tests to diagnose these ‘infectious diseases’ reliably. Let’s remember that a prominent symptom of Covid-19 was being asymptomatic. Let’s reflect on how they would have determined this by having definite positive/negative cases. How would they have determined this? Other ‘infectious diseases’ that would fall under this category is AIDS (HIV positive with no symptoms), hepatitis, herpes, measles, rubella, cholera, Lyme, etc. How is a gold standard test reliably defined and assessed for reliability if ‘infections’ can be asymptomatic?
Method 1 and 3 both rely entirely on understanding what a sick person is, specifically their symptom profiles. However, since diseases have varied symptom profiles based on the individual, how are we to develop a test, and choose a valid and reasonable indicator, or symptom profile? How is it possible to have correct classification of individuals who are definitely healthy, and those who are definitely diseased? Especially if it is possible for there to be asymptomatic cases.
How would one differentiate cases of measles. It is impossible, admittedly, to diagnose based on symptoms, as it can resemble other diseases. How would one differentiate Guillain-Barre Syndrome, from Polio, from Meningitis? An allopath would employ a test. But it begs the question of how would you develop a specific and sensitive test without being able to differentiate these cases from an observational standpoint, if their symptom profiles are indistinguishable, and the methods of assessing the specificity and sensitivity are based on the observational characteristics of a disease?
At this point in the discussion, we can see the invalidity of developing a test surrounding non-empirical agents (viruses, proteins, and antibodies), and realistically tests surrounding any disease in general due to the variability in symptom profiles, hence the need and search for valid tests. Unless there is an empirical observation that can be made in 100% of cases, the creation of a test will be subjective. There is a lack of objectivity in test development. This is a circular process as it relies on other tests, which are validated in the same way.
Nucleic Acid Tests and Sequencing
Here, we will cover the methods used in virology to determine and test for genetic material. You will see that for viruses, their study of genomics is completely in silico, generated by computers. Since viruses are not able to be isolated, these sequences have not been controlled or verified. Viral genomics is largely a circular confirmation bias. Let’s look at some methods involved objectively, and then I will give my comments.
Sanger sequencing
The ‘gold standard’ in genome analysis is Sanger sequencing. Sanger sequencing uses the chain termination method to generate DNA fragments of varying lengths, which are then separated and detected to determine the precise sequence of nucleotides in the original DNA sample. It’s very expensive and a lengthy process. Nowadays, Sanger sequencing is largely used for smaller portions of the genome, such as single genes.
You might see papers that claim to use Sanger sequencing to generate a virus’ genome, but this is not traditional Sanger sequencing. They begin this process by taking primer sequences from a database and ‘extract’ the viral genome through polymerase chain reaction, convert it to complementary DNA strands, and run the Sanger sequencing process.
Comments:
Isolation of the species is required for Sanger sequencing to be a gold standard. Sanger sequencing works better for frogs and humans, as we can isolate that genetic material. Not that there aren’t problems in human genomics, but we will save that discussion for another time. The reason that isolation of genetic material is required for Sanger sequencing to be a gold standard is that it is the only valid way to ensure that the short reads are coming from the species in question.
Let’s remember how viruses are isolated in virology. They are produced by a cell culture. Those cells have genetic material. Therefore, isolation of viral genetic material cannot and has never occurred.
Now, of course, this process of Sanger sequencing used in virology is a form of confirmation bias, as it relies on the PCR primers, which are an uncontrolled endeavor and rely on computational probabilities which we will explore very shortly. Additionally, negative results would be omitted, and only positive results that would have matched the databases would have been accepted. Any variability would have been considered a variant, which we will discuss soon. No new viral genomes are determined using this method, only confirmation.
Next Generation sequencing
Next-generation sequencing (NGS) is a massively parallel sequencing technology that enables the rapid sequencing of DNA or RNA at a much higher throughput compared to traditional Sanger sequencing. It allows for the determination of the order of nucleotides in entire genomes or targeted regions of DNA/RNA. All NGS methods begin with sample preparation.
Viral samples can be obtained from various sources, such as clinical samples, environmental samples, or cell cultures. The samples contain a mixture of viral, bacterial, and host genetic material. Considering RNA viruses, all the DNA would be removed to isolate the genetic material of the virus.
Comments:
There is a misleading attempt to ‘isolate’ the viral genetic material, but this is not a real isolation. Even if they remove the DNA, host cells from clinical samples or cell cultures still contain mRNA, tRNA, rRNA, miRNA, snRNA, etc. Environmental samples contain a plethora of other genetic materials from other sources. Therefore, there is always genetic material contamination. It is important to note here that viral genetic material has never been separated or distinguished from host/environmental genetic material. It is only possible to separate genetic material by the type of genetic material (i.e., isolated DNA or isolated RNA).
A paper might claim to separate the viral genetic material from other genetic material based on the database’s knowledge of previously sequenced viruses, but this is circular reasoning as no viral genome has ever been isolated in the first place. It also begs the question of the existence of a ‘virus’. There is no starting point.
Illumina, PacBio & Nanopore
These are specific examples of next-generation sequencing. These techniques are concerned with cultured samples, which are viruses grown in cell/tissue cultures. An attempt will occur to isolate the viral genetic material. From the genetic material, the next step is sequencing. Here, scientists will use shotgun sequencing technologies like Illumina, PacBio, or Nanopore to generate millions of short sequencing reads from the ‘viral’ genetic material. Next, scientists assemble the sequencing reads into longer contiguous sequences (contigs) representing the full viral genome, of course conducted in silico or computer-generated, which I will cover shortly. They also compare the assembled viral genome to reference databases to determine the taxonomic classification of the novel virus, i.e., which virus family, genus, or species it belongs to.
Comments:
Notably, I want to highlight again that there is an attempt to ‘isolate’ the viral genetic material, but this is not a pure isolation. Host cells still contain mRNA, tRNA, rRNA, miRNA, snRNA, etc. There is always going to be host genetic material contamination. There is no control for this.
Considering the process, the creation of various short reads is the fundamental component of the methodology. But at no point can we truly distinguish viral genetic material from other genetic material. Thus, from a genetic soup, we gather short reads in which we have no understanding of where they are coming from. And assemble them all together.
Again, a confirmation bias occurs when they compare the created viral sequences to the database of genetic sequences. We have never confirmed any genetic material through a properly controlled setup or isolated virus. Another major problem here is the threshold for similar viral material is quite low. SARS-CoV-1 shares 80% of its genome with SARS-CoV-2. For reference, humans and chimps share 98.8% of our genome. So, we think that SARS-CoV-1 and 2 are so closely related, but it would be like comparing humans to cows (which are 80% similar genetically).
Metagenomics (In Silico)
This branch of genetics research is the study of genetic material recovered directly from environmental or clinical samples, without the need to cultivate the organisms first. This technique is applicable to clinical science, soil science, environmental science, marine biology, etc. It is a valid technique when we understand the nature of the genome prior to conducting metagenomic analyses, such as for entities in which we can isolate and use first-generation techniques. The process is the same as highlighted above, but for even less pure samples.
Computer Genomic Sequencing
In silico genomic sequencing is viral genetic sequencing done in computers. It is used to assemble the sequencing reads into longer viral genome sequences, to annotate the viral genomes to identify genomic features and predict protein functions, compare the viral sequences to reference databases to classify them taxonomically, and compare the viral community composition, diversity, and evolution.
Bioinformatic algorithms like de novo assembly or reference-guided assembly are used to piece together the short sequencing reads (small reads of genetic material) into longer contiguous sequences (contigs) representing the full ‘viral genomes’. Scaffolding algorithms can then be used to join the contigs into larger, more ‘complete’ genome sequences. The assembled viral genomes may still have gaps or inconsistencies that require manual review and editing. Tools like ContigExtender, Phables, and Jorg can be used to extend contig ends and join contigs to improve genome completeness.
Computers are based on probabilities. These programs generate millions of sequences as results. The researchers choose the best one that fits their models and expected findings. They also confirm their results by comparing the genetic sequences to other genetic sequences in the database to classify the species.
Comments:
Don’t ask how they control or validate this process without comparing it to other sequences that have been gathered in the same manner. Computer-generated sequences are self-confirmed. They compare computer-generated sequences to other computer-generated sequences to confirm their findings. Indicative of a confirmation bias and circular reasoning. This process has never been controlled and has never been validated. How can we be sure that this is the sequence if we don’t have purified viral genetic material or purified viruses to confirm? Of course, there is subjectivity in this process as well, as researchers have to decide which computer-generated sequence fits their preconceived theories.
Nucleic Acid Testing (Primers)
At this point in the discussion, it is obvious that the creation of a viral genome has never been controlled or confirmed, other than by the methodology used to propose the genome. Thus, the creation of primers is not a controlled or validated endeavor either, as the primers are created based on the genetic sequences that have been computationally created from contaminated samples. Primers are short, single-stranded DNA sequences that are used in various molecular biology techniques, particularly the polymerase chain reaction (PCR).
Polymerase Chain Reaction
PCR is a technique used to rapidly make millions to billions of copies of a specific DNA sequence. Primarily, it is the nucleic acid test used to determine the presence of a virus. For reference, the ‘gold standard’ test for Covid-19 was the PCR test. The key steps in the PCR process are the following.
The DNA sample is heated to high temperatures to separate the double-stranded DNA into single strands. Short DNA sequences called primers, described above, bind to the target DNA region. These primers provide a starting point for the DNA synthesis. An enzyme called DNA polymerase uses the single-stranded DNA as a template to synthesize new complementary DNA strands, starting from the primers. These three steps – denaturation, annealing, and synthesis – are repeated in cycles, typically 25-35 times. With each cycle, the number of DNA copies doubles, leading to exponential amplification of the target sequence.
I was taught in university that it would be inappropriate and scientifically invalid to run a PCR past 33 cycles. In the event of covid, laboratories were running tests from 35-45 cycles. The problem is that with each cycle, there is a chance that an uncomplimentary strand of genetic material might be attached by a primer, leading to a false positive result. The higher the cycle count, the higher the false positive chance.
Let’s also remember back to the section on the determination of specificity and sensitivity of a test. At this point, we have unconfirmed, uncontrolled genetic sequences. The creation of primers based on these sequences. Running experiments to determine the sensitivity and specificity based on these primers, and subjective interpretation of disease symptoms, no gold standard to compare it to, etc. It is obvious that this process is a house of cards. No foundation whatsoever.
Comments:
Largely within genetic sequencing of viruses, there is a lack of control. There is also a lack of gold standard sequencing, such as the use of Sanger sequencing. Viruses are admittedly unable to be isolated in the real sense of the word isolation, which would mean complete separation from all other parts. Isolation in virology refers to viruses being produced in a cell culture, wherein the cells have their own genomic properties. Scientists are largely unable to reliably account for contaminating genetic material from the cell lines that host the alleged viruses. A controlled viral genome analysis has never been conducted. Problematically, to confirm the pseudo-isolation of viruses, they employ genetic tests as we will see in the cell culture portion of this post.
The circle of logic continues here, as they claim to run genomic analysis on ‘isolated’ viruses that were confirmed by the tests created from the genetic sequences determined in silico. The computer-generated sequence was not a controlled endeavor and did not employ the scientific method. Therefore, the genetic test is not a controlled endeavor and did not employ the scientific method. How could this test be scientifically confirmed? We have already established the problems in determining specificity and sensitivity, especially how it is largely based on the rationale of the scientific consensus.
A control that has been claimed to be used in very few viral genomic studies is water devoid of genetic material. Of course, it is evident that comparing taking the snot of an individual or a section of spinal cord, or any sort of fluid or sample from the body is not comparable. A valid control would be to take samples (mucus, tissue, secretions, feces, urine, etc.) from diseased individuals, run the sequencing process, and run the same process on the same type of sample (mucus, tissue, secretions, feces, urine, etc.) gathered from a healthy individual. However, this has never been conducted.
Additionally, just because the process produces similar results doesn’t mean that it is a correct process. The process should be consistent, correct, and accurate. Consistency doesn’t indicate correctness and accuracy. If I shoot a puck three feet wide of the net every single shot, it means that I am rather consistent but completely inaccurate. It is important to note that this process is not necessarily consistent either. Reproducibility in science refers to the process of replicating results exactly, not similarly. For example, with HIV, the genome can have 40% genomic variability and still be considered an HIV ‘virus’. To be clear, the acceptable genomes sequenced in silico only have 60% similar genomic sequences. This is standard across virus genome sequencing.
This points the discussion to that of variants. The variants reported in this case are based on the variation of the results of conducting genomic sequencing. If anything, the concept of variants is indicative of poor consistency within the sequencing techniques used in virology. To truly determine a variant, one would have to isolate the variant from all other viral particles of the same species. Something the consensus would even agree is impossible. A virologist might claim that the variants are present in an individual with a slightly different symptom profile, but again this is rather subjective, based on no scientific evidence, and would end up being circular reasoning, as it begs the question, how was the variant determined to be the causative agent of that distinct subset of symptoms.
Here, we can see that the concept of variants is used to explain the inconsistencies of the genomic sequencing processing. The concept of variants, let alone viral sequencing in general, has never been tested in a controlled manner and makes no attempt at utilizing the scientific method. You can see how scientists can spin these stories based on the observations to fit their established model. Here it would certainly be illogical to point to the scientific consensus backing up this interpretation as the correct one, as we would quickly fall into an ad populum or argumentum ab auctoritate fallacy. We can also see the problems with induction from empirical observation, but I will save that discussion for another time.
Immunological Methods
Antibody research is among the most unreliable and is central to the reproducibility crisis in science. Studies that use antibodies often cannot be reproduced consistently. In this section, we will delve a bit deeper into the immunological methods used in virology specifically and why they are unreliable and largely unscientific.
Antibodies
Antibodies, also known as immunoglobulins, are proteins that identify and neutralize foreign objects like pathogenic bacteria and viruses. They ‘tag’ microbes or infected cells for attack by the immune system or neutralize them directly. Specifically, antibodies bind antigens. Each antibody is said to bind to a specific antigen, which can be a molecule, fragment, or part of a virus, bacteria, or other pathogen. An epitope is a specific region of an antigen that is recognized by the immune system. Antibodies are a major component of the immune system.
There are five main classes of antibodies (IgG, IgM, IgA, IgD, IgE) that have slightly different structures and functions in the body. There are two distinct types of antibodies: monoclonal antibodies, which are highly specific to a single epitope, and polyclonal antibodies, which recognize multiple epitopes on the same antigen. Monoclonal antibodies are said to have minimal cross-reactivity with other proteins, while polyclonal antibodies have a higher chance of cross-reactivity due to binding multiple epitopes. The major difference between monoclonal and polyclonal antibodies is that monoclonal antibodies are a homogeneous mixture, while polyclonal antibodies are a heterogeneous mixture.
Antibody Specificity
Specific antibodies bind to a particular epitope or antigenic site on a pathogen, allowing the immune system to target that specific threat. They are part of the adaptive immune response, produced by B cells in response to a specific antigen. Specific antibodies provide long-lasting, highly targeted immunity against the recognized antigen and exhibit an anamnestic (memory) response upon re-exposure.
On the other hand, non-specific antibodies provide a more general defense against a wide range of pathogens without requiring prior exposure to a specific antigen. They are part of the innate, non-adaptive immune response. Non-specific antibodies often provide a temporary increase in responsiveness, but this effect is not as long-lasting as that of specific antibodies.
How to validate specificity
You should now be asking yourself, what empirical evidence is there for making these assertions. Let’s look at the process of validating antibody specificity. Simply, this process begins with the selection and preparation of positive and negative controls. Positive controls confirm selective antibody binding, while negative controls reveal non-selective binding.
Knockout cell lines that lack the target protein are considered the “gold standard” for assessing antibody specificity. The principle is that if an antibody is truly specific to a target protein, it should show no binding activity in cells where that target protein is absent (i.e., knockout cells). This process relies on removing the genetic sequence and thus relies on our knowledge of genetic sequences. You can use KO cell lines or tissues in all assays: western blot, IHC, ICC, flow cytometry.
The other methods involved in determining positive and negative controls include small interfering RNA (siRNA) knockdown and the manipulation of proteins within a sample. siRNA knockdown involves using post-transcriptional gene silencing. siRNA binds mRNA before the nucleic acids become proteins, minimizing protein expression. Both these methods involve much greater error and limitations. siRNA knockdown relies mostly on our genetic knowledge of the species.
Antibody Tests
It is important to be clear here. Antigen tests differ from antibody tests. Testing for the presence of an antibody in the blood and testing for an antigen in the blood are two distinct procedures. They both rely on the specificity of the antibodies but have different applications. However, this aspect of immunology can be confusing. Sometimes scientists will test for antibodies because their presence indicates the presence of an antigen, which in turn suggests the presence of a virus. At other times, scientists will use antibodies to test directly for a specific protein, or antigen in this case. A secondary antibody is often used to visualize the sample.
Simply put, primary antibodies bind directly to the target antigen or protein of interest. Secondary antibodies bind to the primary antibodies, usually to their Fc region, the fragment crystallizable region, which is the tail region of the antibody structure. Primary antibodies are not typically labeled with a detection tag, so they cannot be directly visualized. Secondary antibodies are often conjugated to enzymes, fluorescent dyes, or other labels to enable detection and visualization of the target.
In the case of SARS-CoV-2, the primary test was the PCR test mentioned earlier, the secondary test was the antigen test. Here the sample (nose swab) was placed into an extraction buffer solution to release the viral antigens. The extracted sample is added to the test device, which contains antibodies specific to the SARS-CoV-2 viral antigens. If the viral antigens are present in the sample, they will bind to these antibodies, producing a colored line on the test device. The known limitations are that antigen tests are generally less sensitive PCR, especially in asymptomatic individuals. It was never concluded that one was infected based on an antigen test, a PCR test was always needed to confirm.
In the SARS-CoV-2 vaccine trials (and all vaccine trials), antibody tests are employed to test the efficacy of vaccines. Antibody levels can serve as “correlates of protection”, which are markers that are associated with vaccine-induced protection against disease. Antibodies in the blood of vaccinated individuals show that the vaccine had an immune response. Ongoing monitoring of antibody levels in vaccinated populations can provide insights into the durability and consistency of vaccine-induced immunity. Elaboration on this topic will occur in other posts.
Let’s now consider HIV. The screening test involves the following. The HIV antibody test is performed on a sample of blood. The test looks for the presence of antibodies to HIV-1 and/or HIV-2. The sample is tested using techniques like enzyme-linked immunosorbent assay (ELISA) or rapid tests to detect the presence of HIV antibodies. If antibodies are detected, the test result is positive, indicating the person has been infected with HIV. If the initial HIV antibody test is positive, the HIV-1/HIV-2 antibody differentiation immunoassay is performed to verify the diagnosis.
Comments:
Regarding SARS-CoV-2, you’ll recall the conflicting results with the PCR test; they seemed random and varied based on the number of cycles the lab was running. I noticed that the antigen test produced positive results for anyone who was ill; any sort of symptom resulted in a positive outcome. This is due to the non-specific nature of the antibodies used to detect the antigen in the test.
It’s interesting how in the case of HIV, the presence of antibodies indicates the presence of disease. This is also true for hepatitis, bacterial infections such as Lyme disease, syphilis, and tuberculosis, as well as parasitic infections like malaria, toxoplasmosis, etc. Antibodies therefore indicate either an active infection or a recent one.
Here we can see yet another example of the challenges in empiricism and induction. The parameters of the study, the research question, and the phenomenon in question dictate the perspective of the researcher. For instance, in a short-term vaccine efficacy study, antibodies indicate a positive vaccine response; whereas in a long-term vaccine efficacy study, antibodies indicate immunity. Similarly, in some infections, antibodies indicate an active infection, while in others, they indicate a previous infection.
Western blot
Let’s discuss western blot in relation to virology. Western blot is a widely used analytical technique in molecular biology and immunogenetics to detect and identify specific proteins in a sample. It is said that western blot allows the detection and identification of specific viral proteins within a complex mixture of proteins. First, ‘purified’ viral particles are separated by size using gel electrophoresis, typically, sodium dodecyl sulfate polyacrylamide gel electrophoresis (SDS-PAGE). Here the viral particles are denatured, as well as the proteins. This is a part of running an SDS-PAGE. SDS-PAGE separates proteins by size, not by type. A reference is used to determine which bands correspond to which size.
After the viral proteins are separated by size in the gel, they are then transferred from the gel onto a membrane, such as nitrocellulose or Polyvinylidene fluoride (PVDF). The membrane with the transferred viral proteins is then exposed to antibodies that are specific to the viral proteins of interest. These primary antibodies bind to the target viral proteins on the membrane. A secondary antibody, often conjugated to an enzyme or fluorescent label, is then added and binds to the primary antibody. This allows the detection and visualization of the specific viral proteins on the membrane.
Largely, the area of concern here is that the use of SDS during the western blot process denatures the native structure of proteins, leading to the loss of conformational epitopes that depend on the 3D structure. This means antibodies that recognize conformational epitopes may not be able to bind to the denatured proteins on the membrane. It may also present unknown epitopes that were hidden by the structure of the protein. Of course, western blot relies on the specificity of antibodies as well.
It is admitted that non-specific binding of antibodies to unrelated proteins or post-translational modifications can lead to false positive signals. Post-translational modifications such as phosphorylation, glycosylation, and acetylation can affect the mobility and immunoreactivity of proteins as well. However, the inconsistent and irreproducible results are also indicative of the cross-reactivity of antibodies, which is the more reasonable induction.
Liquid Phase Assay (ELISA)
Another common method in the antibody world is the Enzyme-Linked Immunosorbent Assay (ELISA). The ELISA technique can be used to detect either antibodies (by using an antigen as the capture protein) or antigens (by using an antibody as the capture protein). ELISA is considered the ‘gold standard’ for many diagnostic tests due to its high sensitivity and specificity. Elaboration on this topic will not prove any benefit for or against the argument. If antibodies are specific, it is reliable. If not, the process is a uncertain.
Comments:
Here we must remember what we learned about determining a gold standard test. We should also recall our method of determining the genome of a virus. We will recall that both these processes involve subjectivity and do not involve controls.
The limitations of knockout cells are many, even beyond virology. Generally, the absence of a signal in a knockout sample indicates that the antibody detects the protein of interest specifically in the wild-type sample. However, this observation does not assure that the antibody will not bind nonspecifically to an unrelated protein in a different sample. A major issue is that far too often, in the knockout samples, you can see a positive result, indicating the unreliable and unspecific nature of antibodies.
Largely, the problem with these antibody tests such as ELISA and Western blot is that they fully rely on the specificity of monoclonal antibodies. If antibodies are not specific, the process becomes rather meaningless. These tests may indicate the presence of a certain protein or antigen, but they don’t indicate that an antibody is specific and that it will only bind to a single epitope.
As a quick example, let’s look back on Edward Jenner’s work on cowpox and smallpox. Jenner was able to provide immunity to smallpox by inoculating individuals with the pus from cowpox lesions. Two distinct diseases with different species of viruses, supposedly… Another interesting phenomenon is that it is said that the smallpox vaccines can even confer ‘cross-immunity’ for monkeypox and all orthopox viruses. What does this say about the specificity of antibodies?
When it comes to the specificity of antibodies, what we have is a story. This idea of the adaptive vs. passive immune system has little empirical observation and evidence to support the claims being made. All antibodies display some sort of cross-reactivity. The evidence points to antibodies being nonspecific. There is no antibody that detects only one epitope. Reliance on this leads to misleading results. This process relies on the other methods.
Reasons for observed non-specificity.
In defense of the modern understanding of immunology, lets explore their reasons for the observed non-specificity of antibodies. It is admitted that the following are the sources of false-positive tests. Samples of cells and tissues may express receptors for the Fc portion. Leading to the antibody binding incorrectly to cells or tissues.
Secondary antibodies are used to detect and amplify the signal of primary antibodies in immunoassays. They are selected based on the species in which the primary antibody was raised. However, some secondary antibodies may exhibit non-specific binding to proteins from other animal species, especially when using polyclonal secondary antibodies. This non-specific binding can result in background noise and false positive signals in assays.
Heterophilic antibodies are antibodies present in human serum that can bind to immunoglobulins from species other than humans. For example, HAMA, HAGA, HASA, and HARA are heterophilic antibodies that can bind to antibodies derived from mice, goats, sheep, and rabbits, respectively. The presence of these antibodies in patient samples can lead to false positive signals in immunoassays, particularly when using antibodies derived from animal sources.
Rheumatoid Factors (RFs) are autoantibodies commonly found in the serum of patients with rheumatoid arthritis. They primarily target the Fc portion of human IgG antibodies. However, RFs can also exhibit cross-reactivity with antibodies from other species, including mouse antibodies used in immunoassays. This cross-reactivity can result in false positive or false negative results in assays, particularly when testing samples from individuals with elevated RF levels.
It is important to note that any condition that increases the levels of antibodies in the blood can cause this cross-reactivity too. These conditions include but are not limited to, Other autoimmune diseases, Chronic infections, Chronic inflammatory conditions, Interstitial lung diseases, Chronic kidney disease, Malignancies (cancers), etc.
Other reasons for the observed non-specificity include surface interaction patches. Surface patches of amino acids on antibodies can lead to non-specific binding to unintended targets. There is also Heteromolecular Phase Separation where the presence of certain molecules like DNA or carbohydrates can induce phase separation of antibodies, leading to non-specific binding and aggregation.
Comments:
Again, here we have constructed a story to fit the observations into our preconceived notions. The observations of antibody research all point to the nonspecific nature of antibodies. However, we construct these stories and explanations to justify why we constantly see nonspecific antibody behavior. If anything should be taken from this section, it is that antibody use is an unreliable method of diagnosing, identifying past infection, identifying immunity, identifying immune response, etc. This is also something that is admitted as the limitations of antibody use in diagnostics and research. The use of antibodies in tests and research is not reliable to draw conclusions from, and at the very least, is not reliable to distinguish a virus from a viral-like particle.
Virus identification (Cell culture)
Antibody tests are used to identify the presence of a virus in clinical samples, cell cultures, and cytopathic effect studies. Largely, antibody tests are employed to confirm the presence of a virus within a sample. This is a confirmatory process, used alongside electron microscopy, genetics, and cytopathic effect studies. Often antibody tests are employed to confirm the presence of a virus or viral protein in a sample before CPE is detected.
Comments:
The use of antibody tests is largely an uncontrolled endeavor as well. In scientific experiments, they often only test one antigen or antibody, rather than testing many to control for the specificity of the antibody. Often, controls are omitted as the antibodies they purchase are said to be specific. Scientists will trust that the antibody they purchase binds the target they are looking for and no other targets. There is also the problem of using polyclonal antibodies in virology, which are admitted to being nonspecific.
To properly control the identification of a viral particle using western blot, we would have to run a properly controlled cell culture, with the addition of antibiotics, etc., purify that sample, run an SDS-PAGE, and then run the western blot. However, this is not done in virology. All antibodies display some degree of cross-reactivity. A specific response or positive test is not indicative of specificity. Virology leans on the field of immunology and the notion that antibodies are specific. However, this is just not the case. It is what we are told, but not the reality. The literature, the observations, do not align with this story.
Reproducibility Crisis
The two major fields that the reproducibility crisis plagues are psychology and medicine. More specifically, all medical studies that include antibodies. This includes cancer research, metabolism, immunology, and cell biology. This is something that is admitted by the scientific community. So much so, that there are many databases created to address the antibody reproducibility crisis, which include the Antibody Registry, ABCD Database, Validated Antibody Database, EuroMAbNet, and various antibody informatics tools. However, the impact of these companies is questionable, because regardless, all antibodies display some sort of cross-reactivity to other protein sites.
Comments:
The reality of the situation once again is that we can not rely on the antibodies to tell us whether a virus is present in a sample. There is no way of determining whether the antibody is binding a theoretical virus, or a virus like particle. Not only does the field of immunology or antibody research require reliance on electron microscopy, as well as genetics, but in and of itself it is completely unreliable with no tract record of being valid. It is foolish and unscientific to rely on antibodies to prove the presence and thus the existence of a virus.
We can see once again that concerning virology, one branch of the unreliable, unscientific field relies on other unreliable and unscientific fields to confirm their findings. The circular reasoning continues.
A note on Contagion
Perhaps you’re now thinking, okay so the methods we’ve used are invalid in proving the existence of a virus. But what about the flu, chickenpox, or any other ‘infectious’ disease? You can observe contagion. Simply put, epidemiological examples such as ‘Aunt Betty went to church and got sick’ are not scientific statements. Epidemiological observations make no attempt to control the observation or isolate the variable. The scientific method is not used, and we should now remember the definition of pseudoscience. For as many anecdotal stories of contagion, there are more stories of the opposite. This is a completely invalid way to argue the position of infectious diseases.
It should be known that many contagion studies have been conducted in the past. All of them either fail to isolate the variable, the virus or germ, or involve more than just the microbe being inoculated into people. They often include a plethora of other chemicals, and in many cases, adjuvants such as aluminum hydroxide, which helps stimulate an immune response. Adjuvants are needed in inoculations from laboratory experiments to vaccines, as the viruses or germs alone are not enough to stimulate an immune reaction.
Another less apparent fact at this point is that most controlled contagion studies are unable to produce the desired results. I can point you to a starting point, Milton J. Rosenau’s Experiments to Determine the Mode of Spread of Influenza (1919). If you are truly interested in looking into this information, over 200 contagion studies have been compiled and analyzed in the book Can You Catch a Cold? by Daniel Roytas.
Koch’s postulates or Rivers’ postulates have never been met. There is no isolate, therefore no method to isolate the variable. This is why virology relies on the four horsemen, namely cytopathic effects, electron microscopy, immunological methods, and nucleic acid detection. These four horsemen depict the end of times in virology, as contagion studies have failed time and time again, so science has resorted to trickery and complications to convince themselves and the public of the existence of a replicant competent infectious particle.
If you are curious about other potential causes of disease, especially for viral diseases in this case, look at the etymology of the word virus from Latin, which literally means ‘slimy liquid, poison’. There are only three causes of disease: toxicities, deficiencies, and traumas (physical or emotional). Elaboration on this topic will occur in future posts.
Conclusion
You might think that when all these methods are considered together, they provide enough evidence to believe in the existence of a virus. However, each method depends on the others, creating a circular reasoning. This entire process is redundant and circular. Despite the extensive chain of logic, it remains circular. I have attempted to simplify it here without creating a strawman, hence the separation of my comments and the methods themselves.
Let’s examine the reality of virology. Viruses cannot be isolated. In virology, isolation means having the virus in a cell culture. Therefore, there is no isolated virus or viral genetic material to verify the findings in immunoassays, contagion studies, or genetic studies. There is no confirmation of any of these results. The controls for cytopathic effects show that the standard methodology used creates ‘viruses’, so studies either omit a control or use an improper one. There is no control for any of the EM methods. Not only is the method uncontrolled and unscientific by nature, as the methods used to set up the procedure are unable to be controlled, but the comparison of EM images of healthy cells compared to stressed cells is completely invalid and unscientific as well.
No scientific interpretations can be drawn from any of these methods by themselves, so they all rely on each other. Virology is a self-confirming field. It is the most intricate form of circular reasoning in history. We are convinced of it due to its complexity, but in reality, it is self-confirmed, self-proclaimed, and self-righteous.
I’ve learned that persuasion often comes from within. If you’re a scientist or doctor, it’s crucial to verify the information presented here for the sake of every patient you’ve treated or will treat. Ensuring accuracy is paramount, as the public trusts your expertise and relies on your guidance for their well-being.
You have noticed that I have not included references in this work except for a select few. Not because this text wasn’t well researched and largely based on the literature itself, but because it would be no more convincing with references. I have given you the tools to do research in this YouTube Playlist, if you are unfamiliar with the process of doing research. I want you to learn to do your own research and not rely on others. For those who already understand the fraudulent and pseudoscientific nature of virology, this post was a refresher, and it is likely that you’ve already done your own research. For scientists and doctors, you understand that the processes highlighted are correct, but are offered an alternative perspective, as well as the logical fallacies present in the popular consensus.
Acknowledgments
I would like to thank those on the forefront of this research, namely, Dr. Mark Bailey, Dr. Sam Bailey, Dr. Tom Cowan, Dr. Andrew Kaufman, Mike Donio, Stefano Scoglio, Jamie Andrews, and many more. All of these individuals have helped me see the problems in virology, and germ theory in general.
Disclaimer
The content provided on this website, podcast or social media, is for informational or educational purposes only. It is not intended to be a substitute for professional medical or psychiatric advice, diagnosis, or treatment. Always seek the advice of your physician or other qualified health provider with any questions you may have regarding a medical condition. Never disregard professional medical advice or delay in seeking it because of something you have read on this website, podcast, social media, or in personal conversations.